Documents
Poster
SpectrumNet: Spectrum-based Trajectory Encode Neural Network for Pedestrian Trajectory Prediction
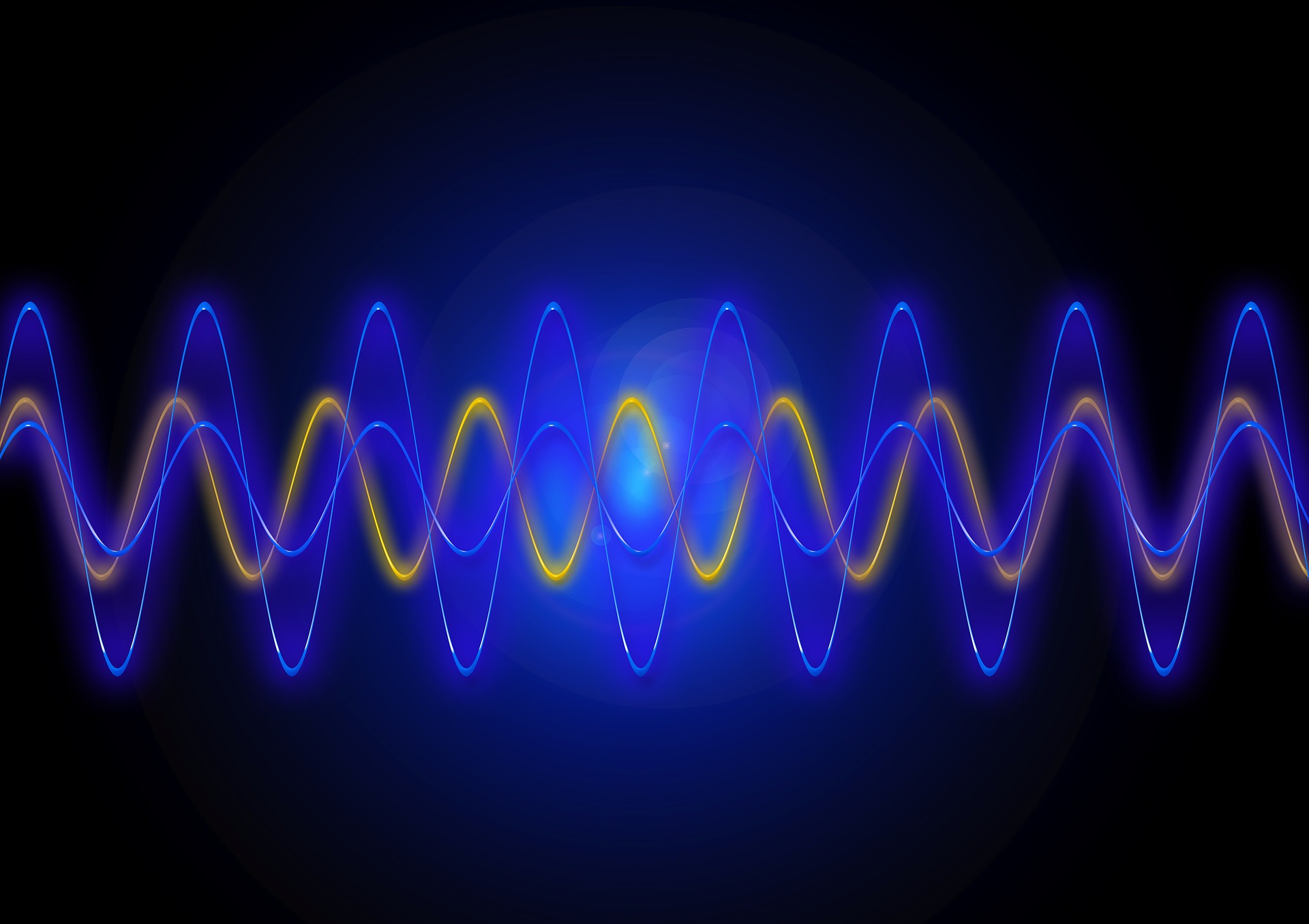
- DOI:
- 10.60864/m0rq-qx52
- Citation Author(s):
- Submitted by:
- Yinglong Zhu
- Last updated:
- 6 June 2024 - 10:32am
- Document Type:
- Poster
- Categories:
- Log in to post comments
Extracting motion pattern implied in the history trajectory is important for the pedestrian trajectory prediction task. The motion pattern determines how a pedestrian moves, including but not limited to reaction of interaction, tendency of speed and direction change. Although the motion pattern is a comprehensive concept and can’t be described concretely, it is clear that it contains both long-term and short-term factors. Inspired by this, we introduce SpectrumNet which enables more effective encoding of historical motion patterns for trajectory prediction. Different from existing methods, which consider the history trajectory as a time sequence of position, SpectrumNet represents it in the frequency space by applying Fourier Transform (FT) to decompose the historical information on different time scales. SpectrumNet consists of two sub-networks, the Multi-Frequency Combination (MFC) encoder, which models the historical information by combining multiple frequency feature in the spectrum; and the Frequency Interaction (FI) encoder, which captures the interaction between pedestrians in the frequency domain. To validate the effect of SpectrumNet, we build a CVAE-based prediction system to predict stochastic future trajectory. Experiments conducted on ETH-UCY dataset show that our prediction system with SpectrumNet out-performs the previous state-of-the-art model and achieves a new record on ADE metric.