Documents
Poster
SPEECH AUGMENTATION USING WAVENET IN SPEECH RECOGNITION
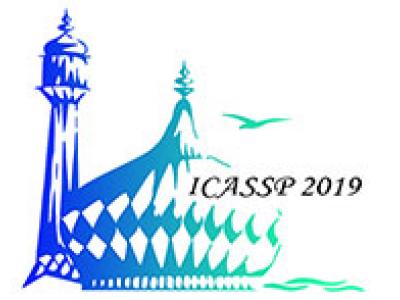
- Citation Author(s):
- Submitted by:
- Jisung Wang
- Last updated:
- 10 May 2019 - 9:55am
- Document Type:
- Poster
- Event:
- Presenters:
- Jisung Wang
- Paper Code:
- SLP-P17.9
- Categories:
- Log in to post comments
Data augmentation is crucial to improving the performance of deep neural networks by helping the model avoid overfitting and improve its generalization. In automatic speech recognition, previous work proposed several approaches to augment data by performing speed perturbation or spectral transformation. Since data augmented in these manners has similar acoustic representations with the original data, it has limited advantage in improving generalization of the acoustic model. In order to avoid generating data with limited diversity, we propose a voice conversion approach using a generative model (WaveNet), which generates a new utterance by transforming an utterance to a given target voice. Our method synthesizes speech with diverse pitch patterns by minimizing the use of acoustic features. With the Wall Street Journal dataset, we verify that our method led to better generalization compared to other data augmentation techniques such as speed perturbation and WORLD-based voice conversion. In addition, when combined with the speed perturbation technique, the two methods complement each other to further improve performance of the acoustic model.