Documents
Presentation Slides
Speech Emotion Recognition Using Deep Neural Network Considering Verbal and Nonverbal Speech Sounds
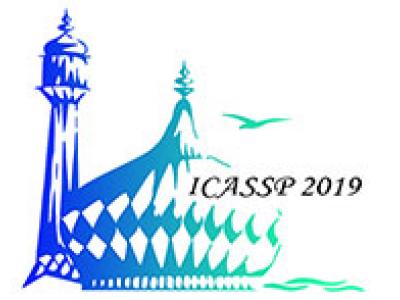
- Citation Author(s):
- Submitted by:
- Chung-Hsien Wu
- Last updated:
- 9 May 2019 - 8:26am
- Document Type:
- Presentation Slides
- Document Year:
- 2019
- Event:
- Presenters:
- Chung-Hsien Wu
- Paper Code:
- ICASSP19005
- Categories:
- Log in to post comments
Speech emotion recognition is becoming increasingly important for many applications. In real-life communication, non-verbal sounds within an utterance also play an important role for people to recognize emotion. In current studies, only few emotion recognition systems considered nonverbal sounds, such as laughter, cries or other emotion interjection, which naturally exists in our daily conversation. In this work, both verbal and nonverbal sounds within an utterance were thus considered for emotion recognition of real-life conversations. Firstly, an SVM-based verbal/nonverbal sound detector was developed. A Prosodic Phrase (PPh) auto-tagger was further employed to extract the verbal/nonverbal segments. For each segment, the emotion and sound features were respectively extracted based on convolutional neural networks (CNNs) and then concatenated to form a CNN-based generic feature vector. Finally, a sequence of CNN-based feature vectors for an entire dialog turn was fed to an attentive LSTM-based sequence-to-sequence model to output an emotional sequence as recognition result. Experimental results on the recognition of seven emotional states in the NNIME (The NTHU-NTUA Chinese interactive multimodal emotion corpus) showed that the proposed method achieved a detection accuracy of 52.00% outperforming the traditional methods.