Documents
Poster
Squared-Loss Mutual Information via High-Dimension Coherence Matrix Estimation
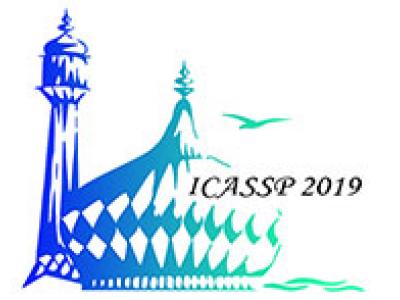
- Citation Author(s):
- Submitted by:
- Ferran de Cabre...
- Last updated:
- 10 May 2019 - 8:02am
- Document Type:
- Poster
- Document Year:
- 2019
- Event:
- Presenters:
- Ferran de Cabrera
- Paper Code:
- SPTM-P4
- Categories:
- Log in to post comments
Squared-loss mutual information (SMI) is a surrogate of Shannon mutual information that is more advantageous for estimation. On the other hand, the coherence matrix of a pair of random vectors, a power-normalized version of the sample cross-covariance matrix, is a well-known second-order statistic found in the core of fundamental signal processing problems, such as canonical correlation analysis (CCA). This paper shows that SMI can be estimated from a pair of independent and identically distributed (i.i.d.) samples as a squared Frobenius norm of a coherence matrix estimated after mapping the data onto some fixed feature space. Moreover, low computation complexity is achieved through the fast Fourier transform (FFT) by exploiting the Toeplitz structure of the involved autocorrelation matrices in that space. The performance of the method is analyzed via computer simulations using Gaussian mixture models.