Documents
Poster
SSL-Net: A Synergistic Spectral and Learning-based Network for Efficient Bird Sound Classification
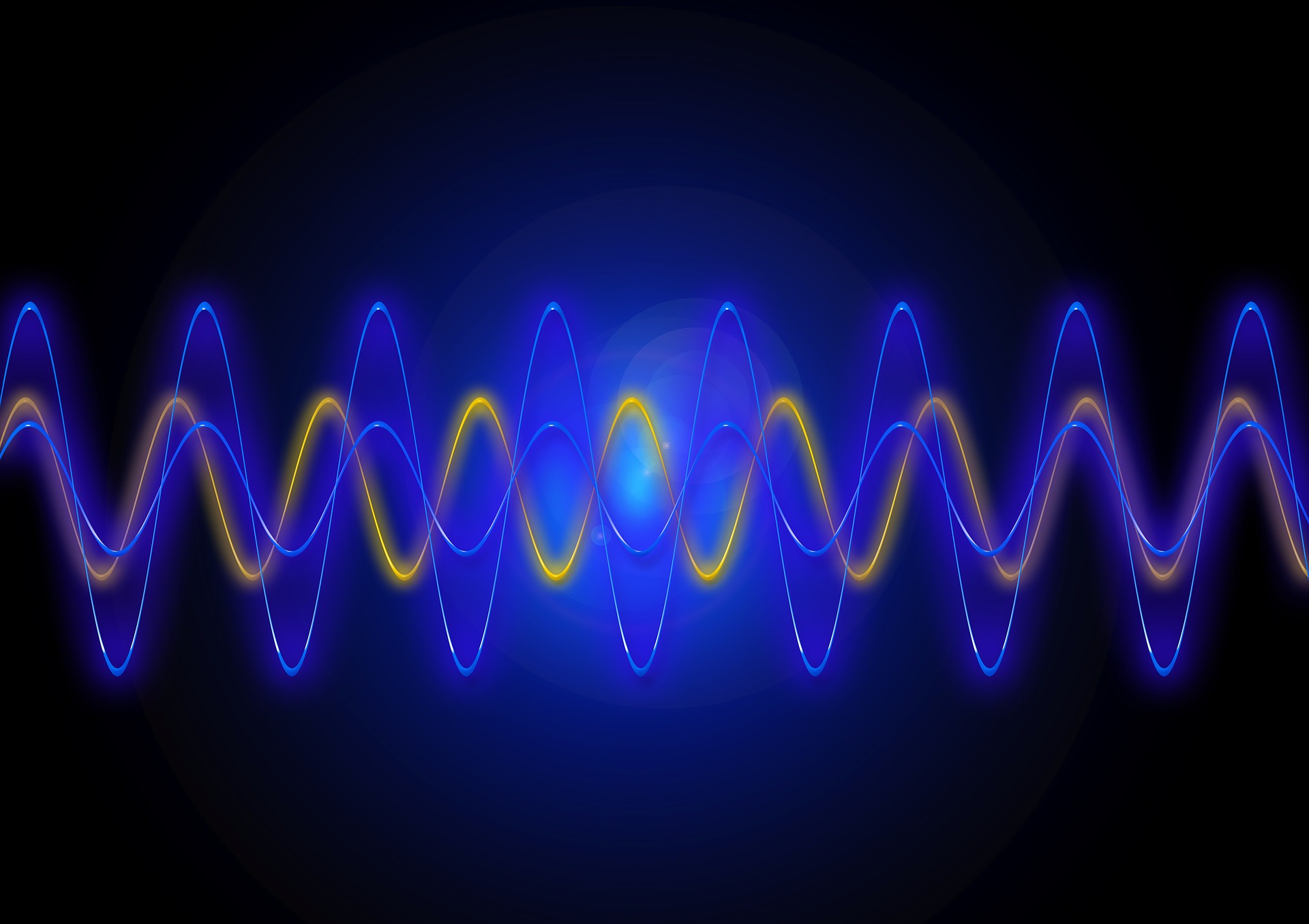
- Citation Author(s):
- Submitted by:
- Yiyuan Yang
- Last updated:
- 2 April 2024 - 7:55pm
- Document Type:
- Poster
- Categories:
- Log in to post comments
Efficient and accurate bird sound classification is of importance for ecology, habitat protection and scientific research, as it plays a central role in monitoring the distribution and abundance of species. However, prevailing methods typically demand extensively labeled audio datasets and have highly customized frameworks, imposing substantial computational and annotation loads. In this study, we present an efficient and general framework called SSL-Net, which combines spectral and learned features to identify different bird sounds. Encouraging empirical results gleaned from a standard field-collected bird audio dataset validate the efficacy of our method in extracting features efficiently and achieving heightened performance in bird sound classification, even when working with limited sample sizes. Furthermore, we present three feature fusion strategies, aiding engineers and researchers in their selection through quantitative analysis.