Documents
Presentation Slides
Stabilizing Multi agent Deep Reinforcement Learning by Implicitly Estimating Other Agents’ Behaviors
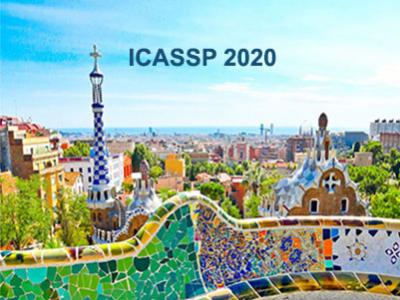
- Citation Author(s):
- Submitted by:
- Yue Jin
- Last updated:
- 17 May 2020 - 8:31am
- Document Type:
- Presentation Slides
- Document Year:
- 2020
- Event:
- Categories:
- Keywords:
- Log in to post comments
Deep reinforcement learning (DRL) is able to learn control policies for many complicated tasks, but it’s power has not been unleashed to handle multi-agent circumstances. Independent learning, where each agent treats others as part of the environment and learns its own policy without considering others’ policies is a simple way to apply DRL to multi-agent tasks. However, since agents’ policies change as learning proceeds, from the perspective of each agent, the environment is non-stationary, which makes conventional DRL methods inefficient. To cope with this challenge, we propose a novel approach where each agent uses an implicit estimate of others’ actions to guide its own policy learning. We demonstrate that given the implicit estimate of others’ actions, each agent can learn its policy in a relatively stationary environment. Extensive experiments show that our method significantly alleviates the non-stationarity and outperforms the state-of-the-art in terms of both convergence speed and policy performance.