Documents
Research Manuscript
Supplementary FMG-Det: Foundation Model Guided Robust Object Detection
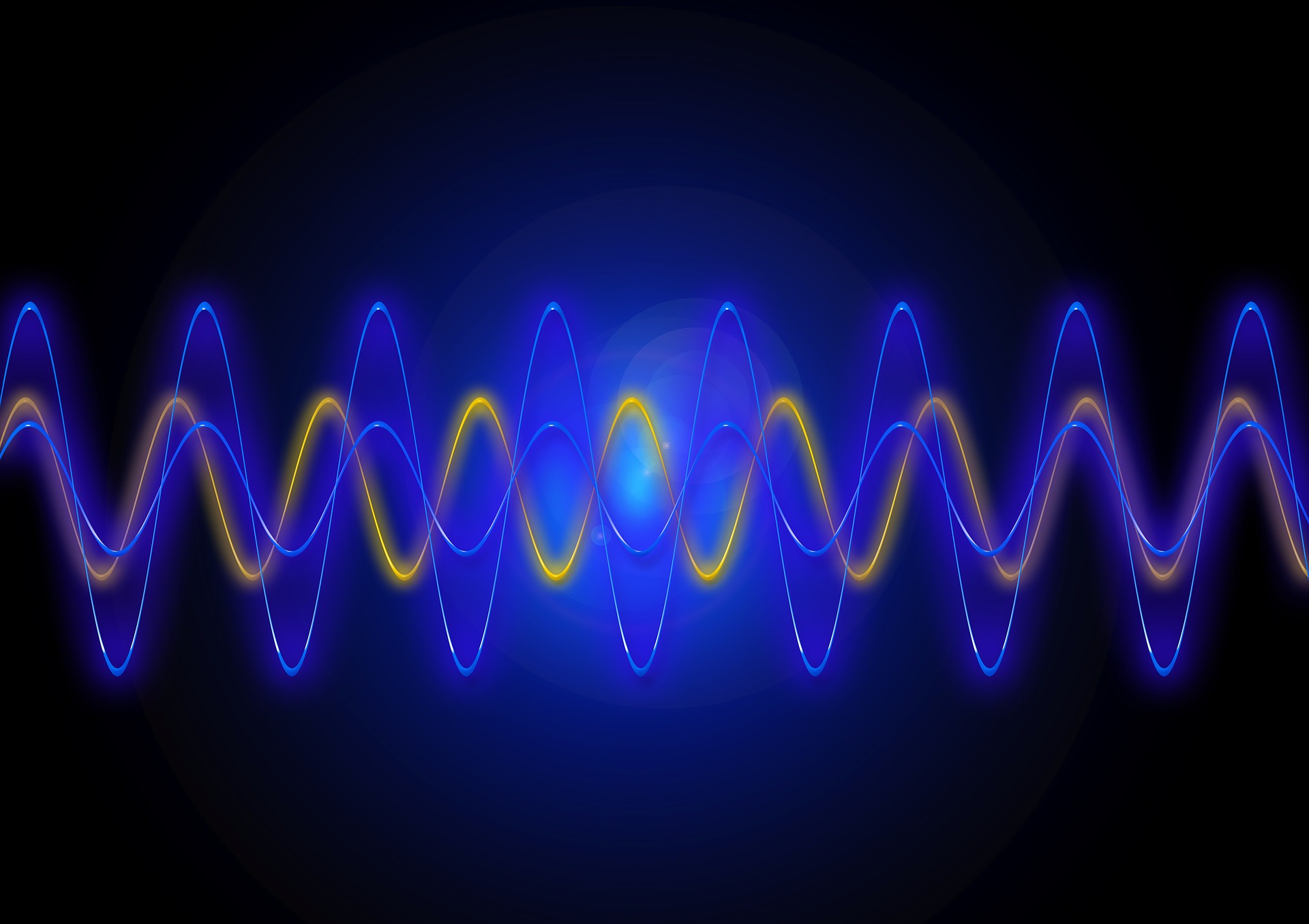
- DOI:
- 10.60864/rd0z-mh96
- Citation Author(s):
- Submitted by:
- Darryl Hannan
- Last updated:
- 14 January 2025 - 10:53pm
- Document Type:
- Research Manuscript
- Categories:
- Log in to post comments
Collecting high quality data for object detection tasks is challenging due to the inherent subjectivity in labeling the boundaries of an object. This makes it difficult to not only collect consistent annotations across a dataset but also to validate them, as no two annotators are likely to label the same object using the exact same coordinates. These challenges are further compounded when object boundaries are partially visible or blurred, which can be the case in many domains. Training on noisy annotations significantly degrades detector performance, rendering them unusable, particularly in few shot settings, where just a few corrupted annotations can impact model performance. In this work, we propose FMG-Det, a simple, efficient methodology for training models with noisy annotations. More specifically, we propose combining a multiple instance learning (MIL) framework with a pre-processing pipeline that leverages powerful foundation models to correct labels prior to training. This pre-processing pipeline, along with slight modifications to the detector head, results in state-of-the-art performance across a number of datasets, for both standard and few-shot scenarios, while being much simpler and more efficient than other approaches.