Documents
Supplementary material
Supplementary material S3VD self-supervised spatial downsampling loss: A method for training video FPN denoising networks
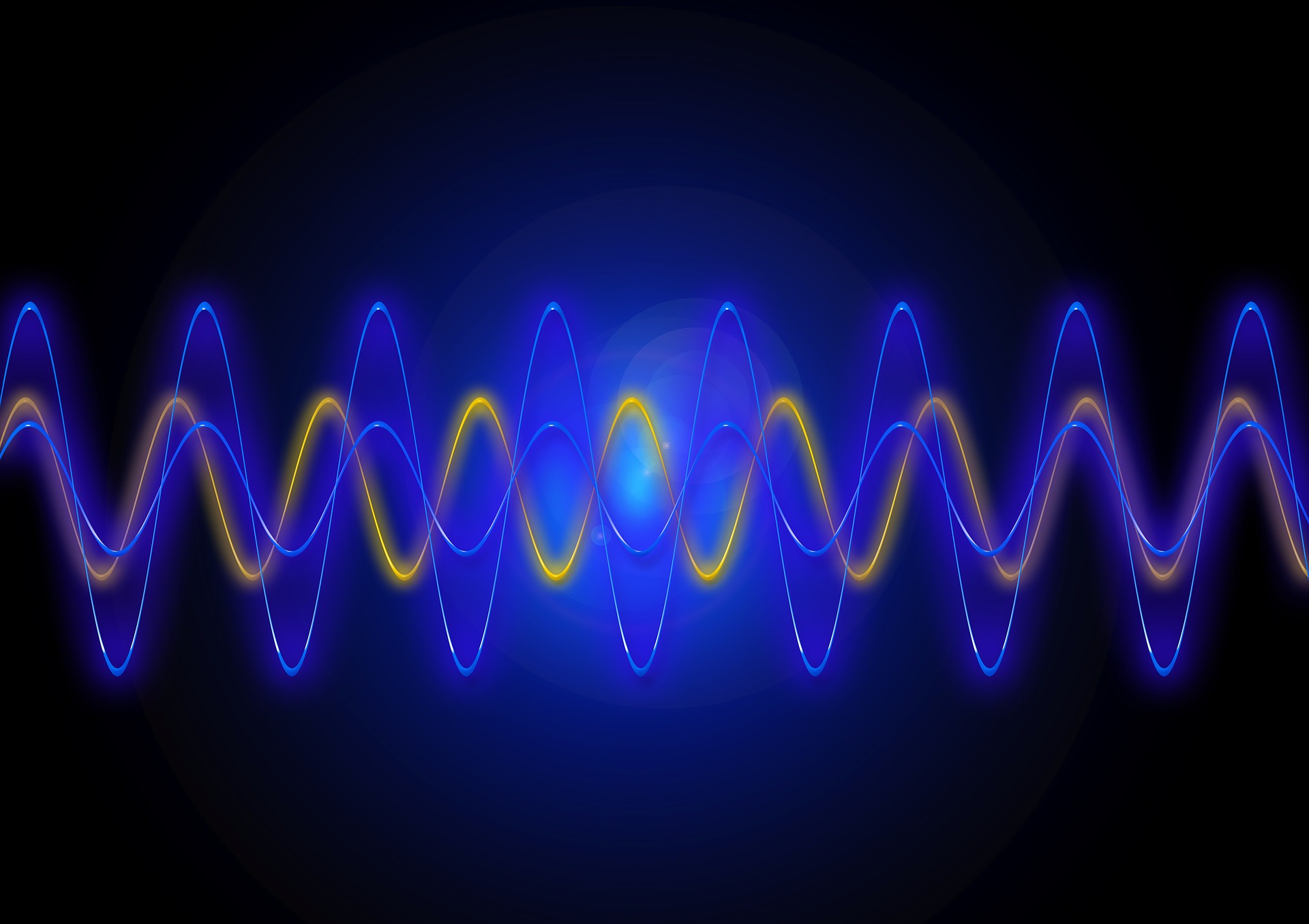
- DOI:
- 10.60864/h8e3-rn93
- Citation Author(s):
- Submitted by:
- Hortensia Barral
- Last updated:
- 5 February 2025 - 7:32am
- Document Type:
- Supplementary material
- Categories:
- Log in to post comments
Fixed pattern noise (FPN) is a temporally constant noise present on videos due to the non-uniformities of the sensors that may exhibit spatial correlation, typically across columns and/or rows.
Acquiring real clean/noisy data is particularly challenging in the case of FPN, leading supervised FPN denoising networks to train using generated data.
Self-supervised approaches for denoising allow training directly on real noisy sequences, avoiding the biases introduced by synthetic data.
However, the spatial and temporal correlation of FPN violates noise independence assumptions underlying most self-supervised approaches.
In this paper, we propose for the first time, a method for training video column FPN denoising networks in a self-supervised way.
Our approach consists of spatial downsampling on rows or columns to obtain quasi-two independent noisy observations from the same images to train a network on.
The proposed method can be applied to any network architecture.
We demonstrate the effectiveness of our method with extensive experiments on synthetic FPN and publicly available real infrared data.