Documents
Supplementary Material
Supplementary Materials of FaceLiVT: Face Recognition using Linear Vision Transformer with Structural Reparameterization
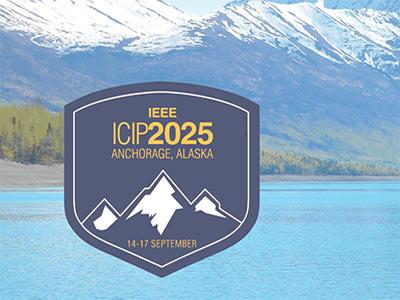
- Citation Author(s):
- Submitted by:
- Novendra Setyawan
- Last updated:
- 6 February 2025 - 6:15am
- Document Type:
- Supplementary Material
- Document Year:
- 2025
- Event:
- Categories:
- Log in to post comments
This paper presents FaceLiVT, a lightweight yet powerful face recognition model that combines a hybrid CNN- Transformer architecture with an innovative and lightweight Multi-Head Linear Attention (MHLA) mechanism. By incorporating MHLA alongside a reparameterized token mixer, FaceLiVT effectively reduces computational complexity while preserving high accuracy. Extensive evaluations on challenging benchmarks—including LFW, CFP-FP, AgeDB-30, IJB-B, and IJB-C—highlight its superior performance compared to state-of-the-art lightweight models. The integration of MHLA significantly enhances inference speed, enabling FaceLiVT to achieve competitive accuracy with lower latency on mobile devices. Notably, FaceLiVT is 8.6× faster than EdgeFace, a recent hybrid CNN-Transformer model optimized for edge devices. With its balanced design, FaceLiVT provides a practical and efficient solution for real-time face recognition on resource-constrained platforms.