Documents
Supplementary Materials: Segment Any Object Model (SAOM)
Supplementary Materials: Segment Any Object Model (SAOM)
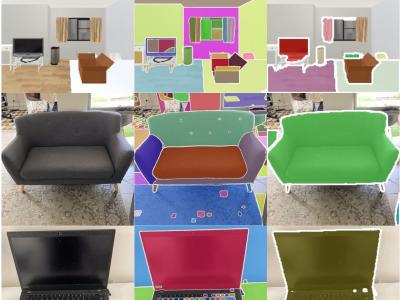
- DOI:
- 10.60864/kqxf-kd78
- Citation Author(s):
- Submitted by:
- Mariia Khan
- Last updated:
- 7 February 2024 - 2:13pm
- Document Type:
- Supplementary Materials: Segment Any Object Model (SAOM)
- Document Year:
- 2024
- Presenters:
- Mariia Khan
- Categories:
- Keywords:
- Log in to post comments
Multi-class multi-instance segmentation is the task of identifying masks for multiple object classes and multiple instances of the same class within an image. The Segment Anything Model (SAM) is a new foundation model designed for promptable multi-class multi-instance segmentation. SAM is able to segment objects in any image using a pre-defined point grid as an input prompt in the ``everything'' mode. However, out of the box SAM tends to output part or sub-part segmentation masks (under-segmentation) in different real-world applications. Whole object segmentation masks play a crucial role for indoor scene understanding, especially in robotics applications. Instead of collecting training data in the real world, we propose a new domain invariant Real-to-Simulation (Real-Sim) fine-tuning strategy for SAM. We use object images and ground truth data collected from Ai2Thor simulator during fine-tuning (real-to-sim). To allow the model work in the ``everything'' mode, we propose the novel nearest neighbour assignment method. We update pre-trained point embeddings from a point grid of the original SAM to each ground-truth mask during the real-to-sim fine-tuning stage. The fine-tuned model, SAOM, can be directly used on real images (sim-to-real) without being previously trained on real-world data. SAOM is evaluated on our own dataset collected from Ai2Thor simulator. SAOM improves on SAM: the mIoU score increases on 28% and mAcc increases on 25% for 54 frequently-seen indoor object classes. Moreover, experimental results show that the proposed Real-Sim fine-tuning strategy has a promising generalization performance in real environments. The dataset and the code will be released after publication.