Documents
Presentation Slides
Symbol-Level Online Channel Tracking for Deep Receivers
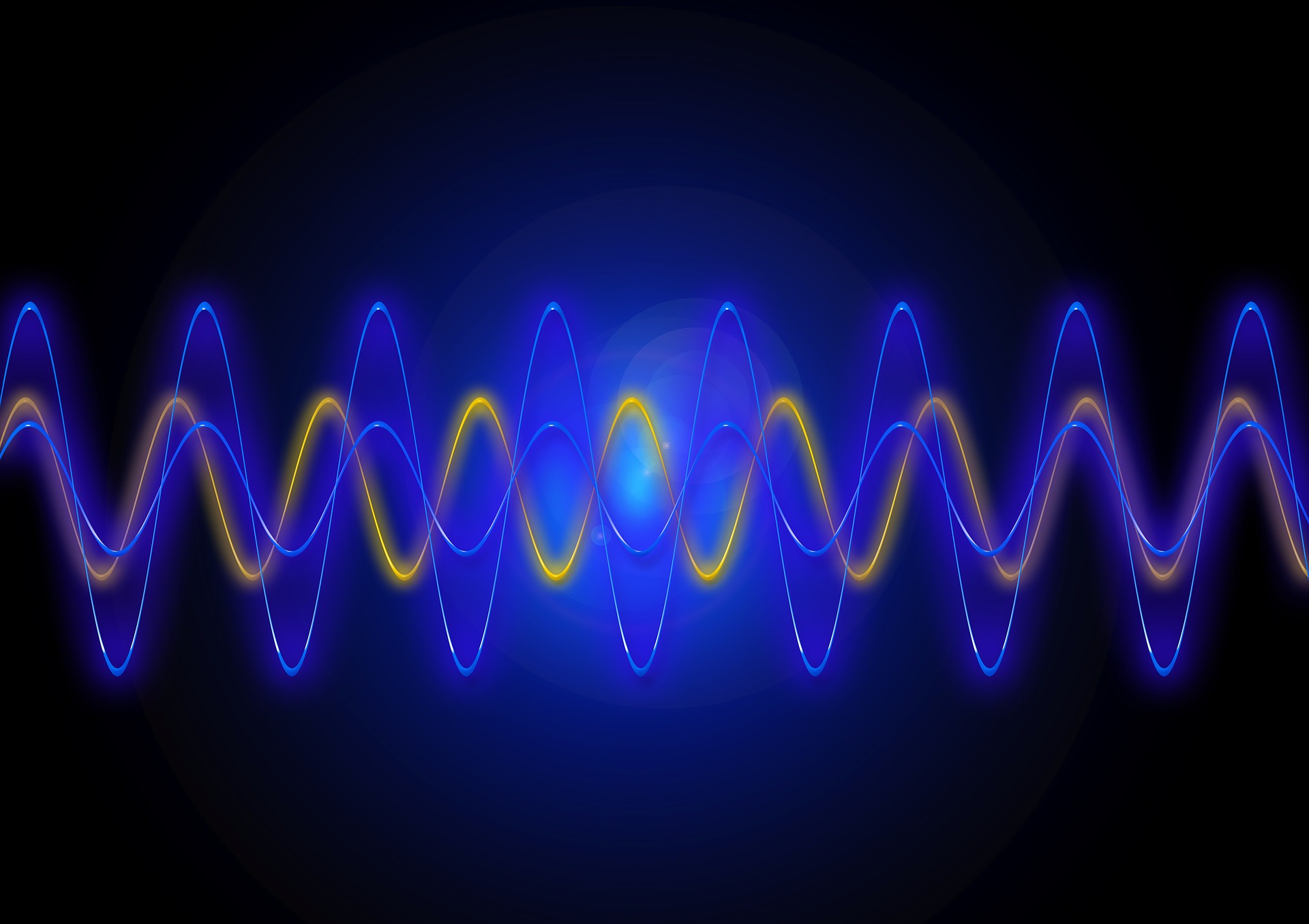
- Citation Author(s):
- Submitted by:
- Tomer Raviv
- Last updated:
- 5 May 2022 - 6:37am
- Document Type:
- Presentation Slides
- Categories:
- Log in to post comments
Deep neural networks (DNNs) allow digital receivers to learn operating in complex environments.
DNNs infer reliably when applied in a similar statistical relationship as the one under which it was trained.
This property constitutes a major drawback of using DNN-aided receivers for dynamic communication systems, whose input-output relationship varies over time.
In such setups, DNN-aided receivers may be required to retrain periodically, which conventionally involves excessive pilot signaling at the cost of reduced spectral efficiency.
In this paper, we study how one can obtain data for retraining deep receivers without sending pilots or relying on specific protocol redundancies, by combining self-supervision with active learning concepts. We focus on the recently proposed ViterbiNet receiver, which integrates into the Viterbi algorithm a DNN for learning the channel. To enable self-supervision, we use the soft-output Viterbi algorithm to evaluate the decision confidence for each of the detected symbols in a given word. Then, to overcome learning with erroneous data, we show how one can choose a subset of the recovered symbols to be used for retraining via active learning. In particular, we propose a method for choosing decision-directed data with a level of confidence that is not too low to result in inaccurate labeling, yet not too high to preserve sufficient diversity of the data.
We demonstrate that this form of self-supervised symbol-level training yields a performance within a small gap of the Viterbi algorithm operating with instantaneous knowledge of the time-varying channel.