Documents
Poster
TAROT: A Hierarchical Framework with Multitask Co-Pretraining on Semi-Structured Data Towards Effective Person-Job Fit
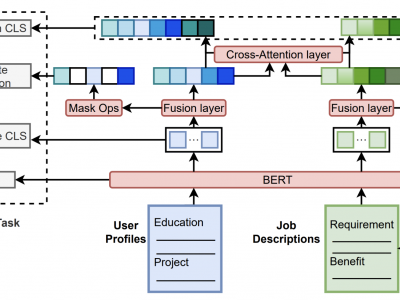
- Citation Author(s):
- Submitted by:
- Yihan Cao
- Last updated:
- 30 March 2024 - 7:25pm
- Document Type:
- Poster
- Document Year:
- 2024
- Event:
- Categories:
- Keywords:
- Log in to post comments
Person-job fit is an essential part of online recruitment platforms in serving various downstream applications like Job Search and Candidate Recommendation. Recently, pretrained large language models have further enhanced the effectiveness by leveraging richer textual information in user profiles and job descriptions apart from user behavior features and job metadata. However, the general domain-oriented design struggles to capture the unique structural information within user profiles and job descriptions, leading to a loss of latent semantic correlations. We propose TAROT, a hierarchical multitask co-pretraining framework, to better utilize structural and semantic information for informative text embeddings. TAROT targets semi-structured text in profiles and jobs, and it is co-pretained with multi-grained pretraining tasks to constrain the acquired semantic information at each level. Experiments on a real-world LinkedIn dataset show significant performance improvements, proving its effectiveness in person-job fit tasks.