Documents
Presentation Slides
Tensor Dictionary Learning with representation quantization for Remote Sensing Observation Compression
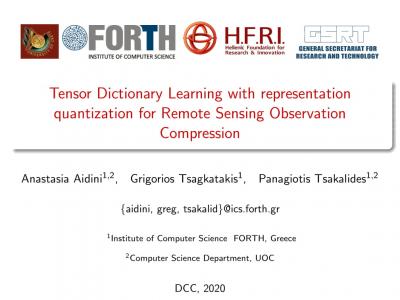
- Citation Author(s):
- Submitted by:
- Anastasia Aidini
- Last updated:
- 30 March 2020 - 8:25am
- Document Type:
- Presentation Slides
- Document Year:
- 2020
- Event:
- Presenters:
- Anastasia Aidini
- Paper Code:
- 176
- Categories:
- Log in to post comments
Nowadays, multidimensional data structures, known as tensors, are widely used in many applications like earth observation from remote sensing image sequences. However, the increasing spatial, spectral and temporal resolution of the acquired images, introduces considerable challenges in terms of data storage and transfer, making critical the necessity of an efficient compression system for high dimensional data. In this paper, we propose a tensor-based compression algorithm that retains the structure of the data and achieves a high compression ratio. Specifically, our method learns a dictionary of specially structured tensors using the Alternating Direction Method of Multipliers, as well as a symbol encoding dictionary. During run-time, a quantized and encoded sparse vector of coefficients is transmitted, instead of the whole multidimensional signal. Experimental results on real satellite image sequences demonstrate the efficacy of our method compared to a state-of-the-art compression method.
dcc_aidini.pdf
