Documents
Poster
A TIME-RESTRICTED SELF-ATTENTION LAYER FOR ASR
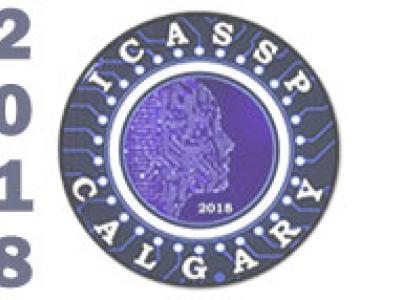
- Citation Author(s):
- Submitted by:
- Hossein Hadian
- Last updated:
- 19 April 2018 - 1:23pm
- Document Type:
- Poster
- Document Year:
- 2018
- Event:
- Paper Code:
- #3560
- Categories:
- Log in to post comments
Self-attention -- an attention mechanism where the input and output
sequence lengths are the same -- has
recently been successfully applied to machine translation, caption generation, and phoneme recognition.
In this paper we apply a restricted self-attention mechanism (with
multiple heads) to speech recognition. By ``restricted'' we
mean that the mechanism at a particular frame only sees input from a
limited number of frames to
the left and right. Restricting the context makes it easier to
encode the position of the input -- we use a 1-hot
encoding of the frame offset. We try introducing
attention layers into TDNN architectures, and replacing LSTM layers
with attention layers in TDNN+LSTM
architectures. We show experiments on a number of ASR
setups. We observe improvements compared to the TDNN and TDNN+LSTM baselines. Attention layers are also faster than LSTM layers in test time, since they lack
recurrence.