Documents
Poster
Towards Building the Federated GPT: Federated Instruction Tuning
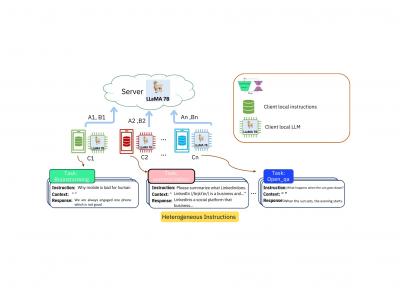
- DOI:
- 10.60864/eh0b-fk89
- Citation Author(s):
- Submitted by:
- Martin Kuo
- Last updated:
- 6 June 2024 - 10:54am
- Document Type:
- Poster
- Document Year:
- 2024
- Event:
- Presenters:
- Martin Kuo
- Paper Code:
- https://github.com/JayZhang42/FederatedGPT-Shepherd
- Categories:
- Log in to post comments
While "instruction-tuned" generative large language models (LLMs) have demonstrated an impressive ability to generalize to new tasks, the training phases heavily rely on large amounts of diverse and high-quality instruction data (such as ChatGPT and GPT-4). Unfortunately, acquiring high-quality instructions, especially when it comes to human-written instructions, can pose significant challenges both in terms of cost and accessibility. Moreover, concerns related to privacy can further limit access to such data, making the process of obtaining it a complex and nuanced undertaking. Consequently, this hinders the generality of the tuned models and may restrict their effectiveness in certain contexts. To tackle this issue, our study introduces a new approach called Federated Instruction Tuning (FedIT), which leverages federated learning (FL) as the learning framework for the instruction tuning of LLMs. This marks the first exploration of FL-based instruction tuning for LLMs. This is especially important since text data is predominantly generated by end users. For example, collecting extensive amounts of everyday user conversations in different languages can be a useful approach to improving the generalizability of LLMs, allowing them to generate authentic and natural responses. Therefore, it is imperative to design and adapt FL approaches to effectively leverage these users’ diverse instructions stored on local devices, while preserving privacy and ensuring data security. In the current paper, by conducting widely used GPT-4 auto-evaluation, we demonstrate that by exploiting the heterogeneous and diverse sets of instructions on the client’s end with the proposed framework FedIT, we improved the performance of LLMs compared to centralized training with only limited local instructions. Further, in this paper, we developed a Github repository named Shepherd. This repository offers a foundational framework for exploring federated fine-tuning of LLMs using heterogeneous instructions across diverse categories. The framework is designed for ease of use, adaptability, and scalability to accommodate large datasets. Additionally, it facilitates the seamless integration of novel algorithms and configurations, making it a convenient tool for researchers and practitioners in the NLP community.