Documents
Poster
Trajectory Optimization for Autonomous Flying Base Station via Reinforcement Learning
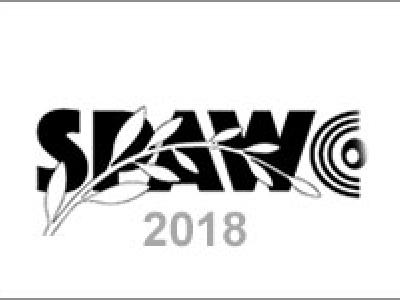
- Citation Author(s):
- Submitted by:
- Harald Bayerlein
- Last updated:
- 3 July 2018 - 10:13am
- Document Type:
- Poster
- Document Year:
- 2018
- Event:
- Presenters:
- Harald Bayerlein
- Categories:
- Log in to post comments
In this work, we study the optimal trajectory of an unmanned aerial vehicle (UAV) acting as a base station (BS) to serve multiple users. Considering multiple flying epochs, we leverage the tools of reinforcement learning (RL) with the UAV acting as an autonomous agent in the environment to learn the trajectory that maximizes the sum rate of the transmission during flying time. By applying Q-learning, a model-free RL technique, an agent is trained to make movement decisions for the UAV. We compare table-based and neural network (NN) approximations of the Q-function and analyze the results. In contrast to previous works, movement decisions are directly made by the neural network and the algorithm requires no explicit information about the environment and is able to learn the topology of the network to improve the system-wide performance.