Documents
Poster
A transfer learning approach to pronunciation scoring
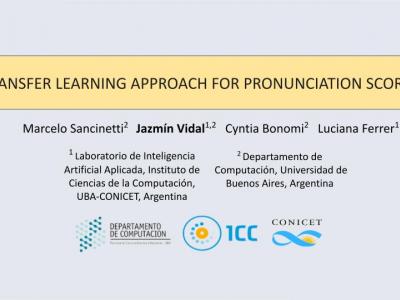
- Citation Author(s):
- Submitted by:
- Jazmin Vidal
- Last updated:
- 8 May 2022 - 12:49pm
- Document Type:
- Poster
- Document Year:
- 2022
- Event:
- Presenters:
- Jazmín Vidal
- Paper Code:
- 3260
- Categories:
- Keywords:
- Log in to post comments
Phone-level pronunciation scoring is a challenging task, with performance far from that of human annotators. Standard systems generate a score for each phone in a phrase using models trained for automatic speech recognition (ASR) with native data only. Better performance has been shown when using systems that are trained specifically for the task using non-native data. Yet, such systems face the challenge that datasets labelled for this task are scarce and usually small. In this paper, we present a transfer learning-based approach that leverages a model trained for ASR, adapting it for the task of pronunciation scoring. We analyze the effect of several design choices and compare the performance with a state-of-the-art goodness of pronunciation (GOP) system. Our final system is 20% better than the GOP system on EpaDB, a database for pronunciation scoring research, for a cost function that prioritizes low rates of unnecessary corrections.
2022-ICASSP (1).pdf
