Documents
Poster
Transform Domain based Medical Image Super-Resolution via Deep Multi-scale Network
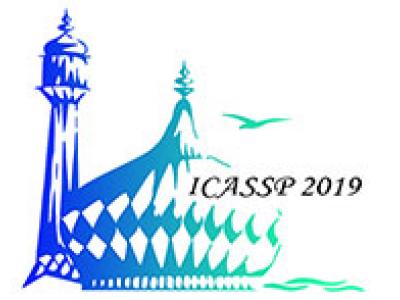
- Citation Author(s):
- Submitted by:
- Chunpeng Wang
- Last updated:
- 8 May 2019 - 9:28pm
- Document Type:
- Poster
- Document Year:
- 2019
- Event:
- Presenters:
- Chunpeng Wang
- Paper Code:
- 4680
- Categories:
- Log in to post comments
This paper proposes a new medical image super-resolution (SR) network, namely deep multi-scale network (DMSN), in the uniform discrete curvelet transform (UDCT) domain. DMSN is made up of a set of cascaded multi-scale fushion (MSF) blocks. In each MSF block, we use convolution kernels of different sizes to adaptively detect the local multiscale feature, and then local residual learning (LRL) is used to learn effective feature from preceding MSF block and current multi-scale features. After obtaining multi-scale features of different MSF block, we use global feature fusion (GFF) to jointly and adaptively learn global hierarchical features in a holistic manner. Finally, compared with other prediction methods in spatial domain, we applied DMSN in UDCT domain, which enables a better representation of global topological structure and local texture detail of HR images. DMSN shows superior performance over other state-of-the-art medical image SR methods.
poster.pdf
