Documents
Poster
TRLS: A TIME SERIES REPRESENTATION LEARNING FRAMEWORK VIA SPECTROGRAM FOR MEDICAL SIGNAL PROCESSING
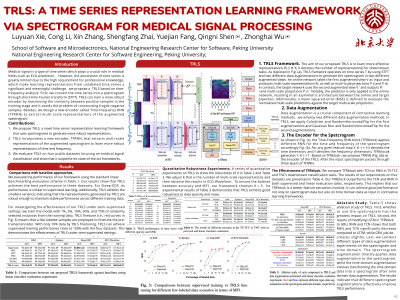
- DOI:
- 10.60864/py2a-1j65
- Citation Author(s):
- Submitted by:
- Luyuan Xie
- Last updated:
- 1 April 2024 - 4:28am
- Document Type:
- Poster
- Document Year:
- 2024
- Event:
- Categories:
- Log in to post comments
Representation learning frameworks in unlabeled time series have been proposed for medical signal processing. Despite the numerous excellent progresses have been made in previous works, we observe the representation extracted for the time series still does not generalize well. In this paper, we present a Time series (medical signal) Representation Learning framework via Spectrogram (TRLS) to get more informative representations. We transform the input time-
domain medical signals into spectrograms and design a time-frequency encoder named Time Frequency RNN (TFRNN) to capture more robust multi-scale representations from the augmented spectrograms. Our TRLS takes spectrogram as input with two types of different data augmentations and maximizes the similarity between positive ones, which effectively circumvents the problem of designing negative samples. Our evaluation of four real-world medical signal datasets focusing on medical signal classification shows that TRLS is superior to the existing frameworks.