Documents
Poster
Two-stage Unsupervised Learning Method for Affine and Deformable Registration
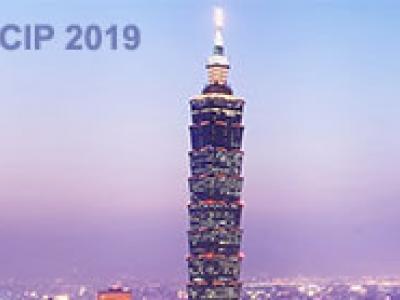
- Citation Author(s):
- Submitted by:
- Dongdong Gu
- Last updated:
- 12 September 2019 - 1:32am
- Document Type:
- Poster
- Document Year:
- 2019
- Event:
- Presenters:
- Dongdong Gu
- Paper Code:
- 1128
- Categories:
- Log in to post comments
Conventional medical image registration relies on time-consuming iterative optimization. We propose a two-stage unsupervised learning method for 3D medical image registration. In the first stage, we learn a global image-wise affine map by a deep network. In the second stage, we learn a local voxel-wise deformation vector field by an encoder-decoder architecture. The final registered image is acquired by applying the local deformation field to the moved image of the first stage. The two networks are trained in an unsupervised manner by maximizing global and local normalized cross-correlations between the fixed image and moved images in the two stages respectively. The well-trained networks can be directly adopted to register images through forward propagation without iteration. We do not need ground-truth in training stage and aligning images in preprocess step. Experiments on four brain MRI datasets demonstrate that the proposed approach outperforms several state-of-the-art methods in terms of accuracy and efficiency.