Documents
Presentation Slides
Unconstrained Flood Event Detection Using Adversarial Data Augmentation
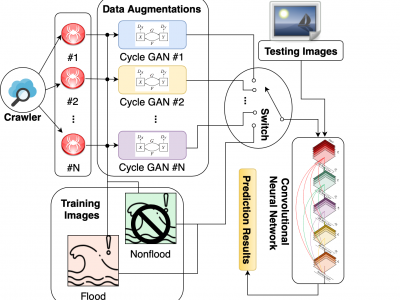
- Citation Author(s):
- Submitted by:
- Yudong Tao
- Last updated:
- 18 September 2019 - 10:55am
- Document Type:
- Presentation Slides
- Document Year:
- 2019
- Event:
- Presenters:
- Yudong Tao
- Paper Code:
- MA.L6.2
- Categories:
- Log in to post comments
Nowadays, the world faces extreme climate changes, resulting in an increase of natural disaster events and their severities. In these conditions, the necessity of disaster information management systems has become more imperative. Specifically, in this paper, the problem of flood event detection from images with real-world conditions is addressed. That is, the images may be taken in several conditions, including day, night, blurry, clear, foggy, rainy, different lighting conditions, etc. All these abnormal scenarios significantly reduce the performance of the learning algorithms. In addition, many existing image classification methods use datasets that usually include high-resolution images without considering real-world noise. In this paper, we propose a new image classification framework based on adversarial data augmentation and deep learning algorithms to address the aforementioned problems. We validate the performance of the flood event detection framework on a real-world noisy visual dataset collected from social networks.