Documents
Presentation Slides
UNIT-DSR: DYSARTHRIC SPEECH RECONSTRUCTION SYSTEM USING SPEECH UNIT NORMALIZATION
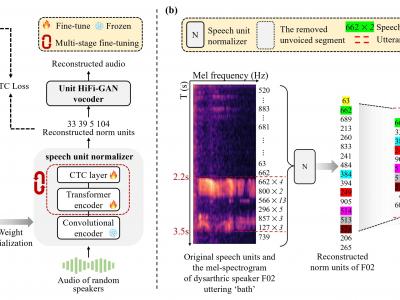
- DOI:
- 10.60864/nbqq-jr10
- Citation Author(s):
- Submitted by:
- yuejiao wang
- Last updated:
- 6 June 2024 - 10:21am
- Document Type:
- Presentation Slides
- Document Year:
- 2024
- Event:
- Presenters:
- Yuejiao WANG
- Paper Code:
- SLP-L2.5
- Categories:
- Log in to post comments
Dysarthric speech reconstruction (DSR) systems aim to automatically convert dysarthric speech into normal-sounding speech. The technology eases communication with speakers affected by the neuromotor disorder and enhances their social inclusion. NED-based (Neural Encoder-Decoder) systems have significantly improved the intelligibility of the reconstructed speech as compared with GAN-based (Generative Adversarial Network) approaches, but the approach is still limited by training inefficiency caused by the cascaded pipeline and auxiliary tasks of the content encoder, which may in turn affect the quality of reconstruction. Inspired by self-supervised speech representation learning and discrete speech units, we propose a Unit-DSR system, which harnesses the powerful domain-adaptation capacity of HuBERT for training efficiency improvement and utilizes speech units to constrain the dysarthric content restoration in a discrete linguistic space. Compared with NED approaches, the Unit-DSR system only consists of a speech unit normalizer and a Unit HiFi-GAN vocoder, which is considerably
simpler without cascaded sub-modules or auxiliary tasks. Results on the UASpeech corpus indicate that Unit-DSR outperforms competitive baselines in terms of content restoration, reaching a 28.2% relative average word error rate reduction when compared to original dysarthric speech, and shows robustness against speed perturbation and noise.
.
Comments
UNIT-DSR: DYSARTHRIC SPEECH
UNIT-DSR: DYSARTHRIC SPEECH RECONSTRUCTION SYSTEM USING SPEECH UNIT NORMALIZATION