Documents
Poster
Unpaired Image-to-Image Translation from Shared Deep Space
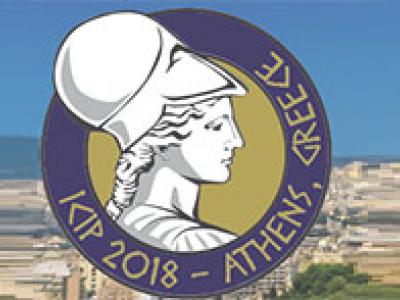
- Citation Author(s):
- Submitted by:
- Jie Shao
- Last updated:
- 4 October 2018 - 9:44am
- Document Type:
- Poster
- Document Year:
- 2018
- Event:
- Presenters:
- Jie Shao
- Paper Code:
- 1177
- Categories:
- Log in to post comments
Unpaired image-to-image translation is a tricky task which aims at learning a mapping from one image collection to another image collection without any pair-labeled information. Recent works have proposed cycle-consistency assumption to deal with this task. However, the result is still unsatisfactory for geometric translation. To address this limitation, this paper proposes a novel method using shared deep space generative adversarial network (SDSGAN). Both two images are encoded into a shared deep space through a pre-trained VGG-19 network, and then we use two decoders to convert them separately to corresponding image domains. In addition, we introduce skip-connection block and self-reconstruction loss to facilitate the mapping. Experimental results show that the proposed SDSGAN has both numerical and perceptual superiorities to existing methods.