Documents
Presentation Slides
Unsupervised learning from limited available data by β-NMF and dual autoencoder
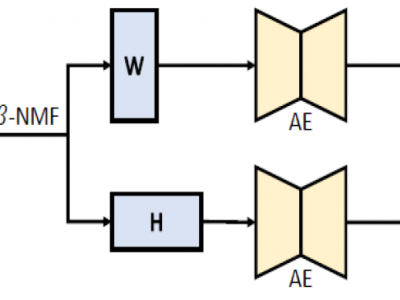
- Citation Author(s):
- Submitted by:
- Angelo Genovese
- Last updated:
- 6 November 2020 - 4:26am
- Document Type:
- Presentation Slides
- Document Year:
- 2020
- Event:
- Presenters:
- Mohanad Abukmeil
- Paper Code:
- SMR-03.5
- Categories:
- Log in to post comments
Unsupervised Learning (UL) models are a class of Machine Learning (ML) which concerns with reducing dimensionality, data factorization, disentangling and learning the representations among the data. The UL models gain their popularity due to their abilities to learn without any predefined label, and they are able to reduce the noise and redundancy among the data samples. However, generalizing the UL models for different applications including image generation, compression, encoding, and recognition faces different challenges due to limited available data for learning, diversity, and complex dimensions. To overcome such challenges, we propose a partial learning procedure by utilizing the β-Non Negative Matrix Factorization (β-NMF), which maps the data into two complementary subspaces constituting generalized driven priors among the data. Moreover, we employ a dual-shallow Autoencoder (AE) to learn the subspaces separately or jointly for image reconstruction and visualization tasks, where our model performance shows superior results to the literary works when learning the model with a small amount of data and generalizing it for large-scale unseen data.