Documents
Poster
Unsupervised Speech Enhancement with speech recognition embedding and disentanglement losses
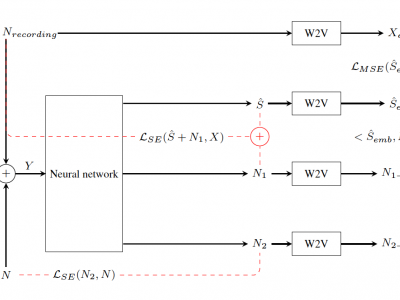
- Citation Author(s):
- Submitted by:
- Viet Anh Trinh
- Last updated:
- 5 May 2022 - 1:31am
- Document Type:
- Poster
- Document Year:
- 2022
- Event:
- Presenters:
- Viet Anh Trinh
- Paper Code:
- AUD-11.6
- Categories:
- Log in to post comments
Speech enhancement has recently achieved great success with various
deep learning methods. However, most conventional speech enhancement
systems are trained with supervised methods that impose two
significant challenges. First, a majority of training datasets for speech
enhancement systems are synthetic. When mixing clean speech and
noisy corpora to create the synthetic datasets, domain mismatches
occur between synthetic and real-world recordings of noisy speech
or audio. Second, there is a trade-off between increasing speech
enhancement performance and degrading speech recognition (ASR)
performance. Thus, we propose an unsupervised loss function to
tackle those two problems. Our function is developed by extending
the MixIT loss function with speech recognition embedding and
disentanglement loss. Our results show that the proposed function
effectively improves the speech enhancement performance compared
to a baseline trained in a supervised way on the noisy VoxCeleb
dataset. While fully unsupervised training is unable to exceed the
corresponding baseline, with joint super- and unsupervised training,
the system is able to achieve similar speech quality and better ASR
performance than the best supervised baseline.