Documents
Presentation Slides
Video Transformer based Video Quality Assessment with Spatiotemporally adaptive Token Selection and Assembly
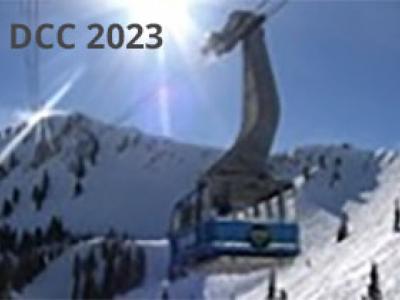
- Citation Author(s):
- Submitted by:
- Shiling Zhao
- Last updated:
- 26 February 2023 - 2:55am
- Document Type:
- Presentation Slides
- Document Year:
- 2023
- Event:
- Presenters:
- ShilingZhao
- Paper Code:
- DCC 244
- Categories:
- Log in to post comments
Video quality assessment (VQA) for user generated content (UGC) videos plays important role in video compression and processing. Convolutional neural network (CNN) based quality assessment for UGC is the research focus with inspiring model accuracy increment in the past three years. However, regularly temporal-sampling with temporal feature loss, as well as fixed token selection strategy video transformer (ViT) with insufficient representational capacity of tokens, jointly degrade the accuracy of conventional ViT based quality assessment. Facing these two challenges, this article proposes an adaptive token-selection ViT (ATSViT) structure for UGC VQA. Accounting for the uneven distribution of spatiotemporal distortion-related features, this work proposes a timing block sampling (TBS) module to adaptively select video blocks and assemble them into content compacted subsequence for further processing. In addition, inspired by the mental filter theory in terms of visual information, we propose a stage-wise adaptive screening network (SSNet) in which “noise” features of tokens in the sense of perception are progressively detected and processed by imitating the behavior of perception process in the eye-brain system. Experimental results verify that the proposed VQA model achieves state-of-the-art (SOTA) accuracy, with the highest correlation with mean opinion scores (MOS).