Documents
Presentation Slides
VOICE BASED CLASSIFICATION OF PATIENTS WITH AMYOTROPHIC LATERAL SCLEROSIS, PARKINSON'S DISEASE AND HEALTHY CONTROLS WITH CNN-LSTM USING TRANSFER LEARNING
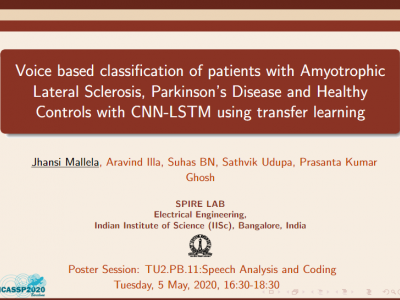
- Citation Author(s):
- Submitted by:
- Jhansi Mallela
- Last updated:
- 26 May 2020 - 1:39am
- Document Type:
- Presentation Slides
- Document Year:
- 2020
- Event:
- Presenters:
- Jhansi Mallela
- Paper Code:
- 4923
- Categories:
- Log in to post comments
In this paper, we consider 2-class and 3-class classification problems for classifying patients with Amyotropic Lateral Sclerosis (ALS), Parkinson’s Disease (PD) and Healthy Controls (HC) using a CNN-LSTM network. Classification performance is examined for three different tasks, namely, Spontaneous speech (SPON), Diadochoki-netic rate (DIDK) and Sustained Phonation (PHON). Experiments are conducted using speech data recorded from 60 ALS, 60 PD and60 HC subjects. Classification using SVM and DNN are considered baseline schemes. Classification accuracy of ALS and HC (indicated by ALS/HC) using CNN-LSTM is found to be 89.20% for the PHON task as compared to 78.80% using the best of the base-line schemes. Furthermore, the CNN-LSTM network achieves the highest PD/HC classification accuracy of 88.5% for SPON task and the highest 3-class (ALS/PD/HC) classification accuracy of 85.24% for DIDK task. Experiments using transfer learning at low resource training data show that data from ALS benefits PD/HC classification and vice-versa. Experiments with fine-tuning weights of 3-class (ALS/PD/HC) classifier for 2-class classification (PD/HC or ALS/HC) gives an absolute improvement of 2% classification accuracy in SPON task when compared with randomly initialized 2-class classifier.