Documents
Presentation Slides
WEIGHTED GENERALIZED MEAN POOLING FOR DEEP IMAGE RETRIEVAL
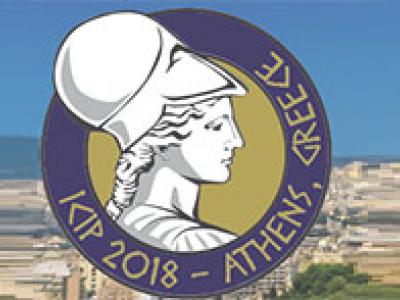
- Citation Author(s):
- Submitted by:
- Xiaomeng Wu
- Last updated:
- 4 October 2018 - 10:08pm
- Document Type:
- Presentation Slides
- Document Year:
- 2018
- Event:
- Presenters:
- Xiaomeng Wu
- Paper Code:
- TP.L2.4
- Categories:
- Log in to post comments
Spatial pooling over convolutional activations (e.g., max pooling or sum pooling) has been shown to be successful in learning deep representations for image retrieval. However, most pooling techniques assume that every activation is equally important, and as a result they suffer from the presence of uninformative image regions that play a negative role as regards matching or lead to the confusion of particular visual instances. To address this issue, we propose a trainable building block that steers pooling to local information important to the task at hand. The method formulates pooling as a weighted generalized mean (wGeM), in which weights are learned on activations, reflecting the discriminative power of each activation in image matching. Embedding wGeM in a deep network improves image representation and boosts retrieval performance on standard benchmarks. wGeM does not require any bounding box annotations, but instead learns the latent probabilities of activations from scratch. It even goes beyond objectness, and learns to look at important visual details rather than the whole region of the object of interest.
TP.L2.4.pdf
