Documents
Poster
Yedrouj-Net: An efficient CNN for spatial steganalysis Results Conclusions • An efficient approach based on deep learning (CNN) for steganalysis. • Our method outperforms the state-of-the-art and others CNN-based models with and without taking extra measu
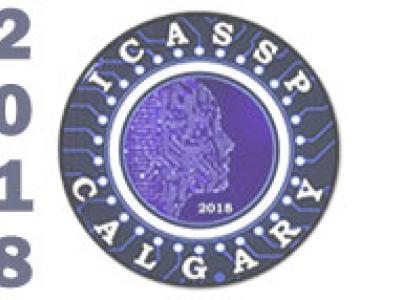
- Citation Author(s):
- Submitted by:
- Mehdi Yedroudj
- Last updated:
- 12 April 2018 - 11:57am
- Document Type:
- Poster
- Document Year:
- 2018
- Event:
- Presenters:
- Yedroudj Mehdi
- Paper Code:
- IFS-P3.3
- Categories:
- Log in to post comments
For about 10 years, detecting the presence of a secret message hidden
in an image was performed with an Ensemble Classifier trained
with Rich features. In recent years, studies such as Xu et al. have
indicated that well-designed Convolutional Neural Networks(CNN)
can achieve comparable performance to the two-step machine learning
approaches.
In this paper we propose a CNN that outperforms the state-ofthe-
art in terms of error probability. The proposition is in the continuity
of what has been recently proposed and it is a clever fusion
of important bricks used in various papers. Among the essential
parts of the CNN, one can cite the use of a pre-processing filterbank
and a Truncation activation function, five convolutional layers
with a Batch Normalization associated with a Scale Layer, as well as
the use of a sufficiently sized fully connected section. An augmented
database has also been used to improve the training of the CNN.
Our CNN was experimentally evaluated against S-UNIWARD
and WOW embedding algorithms and its performances were compared
with those of three other methods: an Ensemble Classifier plus
a Rich Model, and two other CNN steganalyzers.