Documents
Presentation Slides
Supervised group nonnegative matrix factorisation with similarity constraints and applications to speaker identification
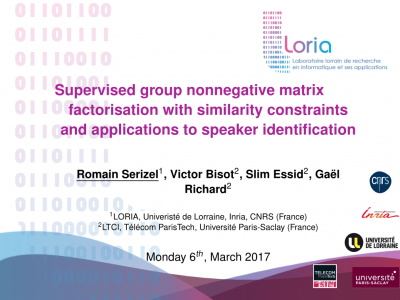
- Citation Author(s):
- Submitted by:
- romain serizel
- Last updated:
- 1 March 2017 - 4:34am
- Document Type:
- Presentation Slides
- Document Year:
- 2017
- Event:
- Presenters:
- Romain Serizel
- Paper Code:
- AASP-L2.2
- Categories:
- Log in to post comments
This paper presents supervised feature learning approaches for speaker identification that rely on nonnegative matrix factorisation. Recent studies have shown that group nonnegative matrix factorisation and task-driven supervised dictionary learning can help performing effective feature learning for audio classification problems.
This paper proposes to integrate a recent method that relies on group nonnegative matrix factorisation into a task-driven supervised framework for speaker identification. The goal is to capture both the speaker variability and the session variability while exploiting the
discriminative learning aspect of the task-driven approach. Results on a subset of the ESTER corpus prove that the proposed approach can be competitive with I-vectors.
Code available at https://github.com/rserizel/TGNMF