Documents
Poster
UNSUPERVISED FEATURE SELECTION BY MANIFOLD REGULARIZED SELF-REPRESENTATION
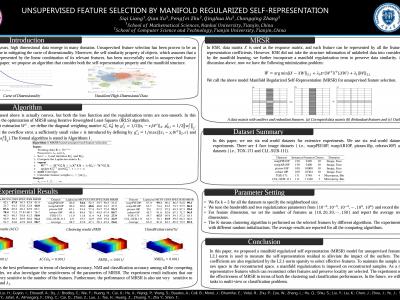
- Citation Author(s):
- Submitted by:
- Qian Xu
- Last updated:
- 13 September 2017 - 12:13am
- Document Type:
- Poster
- Document Year:
- 2017
- Event:
- Presenters:
- Qian Xu
- Paper Code:
- 1266
- Categories:
- Keywords:
- Log in to post comments
Unsupervised feature selection has been proven to be an efficient technique in mitigating the curse of dimensionality. It helps to understand and analyze the prevalent high-dimensional unlabeled data. Recently, based on the self-similarity property of objects, self-representation which assumes that a feature can be represented by the linear combination of its relevant features has been successfully used in unsupervised feature selection. In this paper, we propose a novel algorithm termed Manifold Regularized Selfrepresentation(MRSR) based on the self-representation ability of features. The L2;1-norm constraint is imposed on representation coefficient matrix to select the most discriminant features and remove the irrelevant ones simultaneously. We further incorporate the effective manifold regularization term into MRSR to ensure that features can be represented at utmost with minimum representation residual. Extensive experimental results on several benchmark datasets validate the effectiveness of our method.