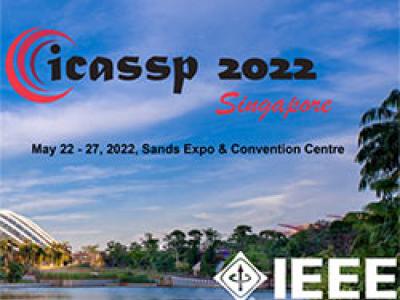
In this paper, a novel multi-head multi-layer perceptron (MLP) structure is presented for implicit neural representation (INR). Since conventional rectified linear unit (ReLU) networks are shown to exhibit spectral bias towards learning low-frequency features of the signal, we aim at mitigating this defect by taking advantage of local structure of the signals. To be more specific, an MLP is used to capture the global features of the underlying generator function of the desired signal.
- Categories:
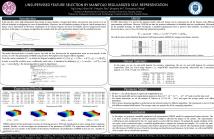
- Read more about UNSUPERVISED FEATURE SELECTION BY MANIFOLD REGULARIZED SELF-REPRESENTATION
- Log in to post comments
Unsupervised feature selection has been proven to be an efficient technique in mitigating the curse of dimensionality. It helps to understand and analyze the prevalent high-dimensional unlabeled data. Recently, based on the self-similarity property of objects, self-representation which assumes that a feature can be represented by the linear combination of its relevant features has been successfully used in unsupervised feature selection. In this paper, we propose a novel algorithm termed Manifold Regularized Selfrepresentation(MRSR) based on the self-representation ability of features.
- Categories: