Documents
Poster
Augmented Lagrangian without alternating directions: practical algorithms for inverse problems in imaging
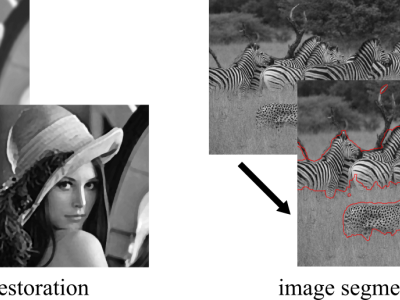
- Citation Author(s):
- Submitted by:
- Rahul Mourya
- Last updated:
- 23 February 2016 - 1:38pm
- Document Type:
- Poster
- Event:
- Presenters:
- Rahul Mourya
- Categories:
- Log in to post comments
Several problems in signal processing and machine learning can be casted as optimization problems. In many cases, they are of large-scale, nonlinear, have constraints, and nonsmooth in the unknown parameters. There exists plethora of fast algorithms for smooth convex optimization, but these algorithms are not readily applicable to nonsmooth problems, which has led to a considerable amount of research in this direction. In this paper, we propose a general algorithm for nonsmooth bound-constrained convex optimization problems. Our algorithm is instance of the so-called augmented Lagrangian, for which theoretical convergence is well established for convex problems. The proposed algorithm is a blend of superlinearly convergent limited memory quasi-Newton method, and proximal projection operator. The initial promising numerical results for total-variation based image deblurring show that they are as fast as the best existing algorithms in the same class, but with fewer and less sensitive tuning parameters, which makes a huge difference in practice.