Documents
Presentation Slides
Discriminative Probabilistic Framework for Generalized Multi-Instance Learning
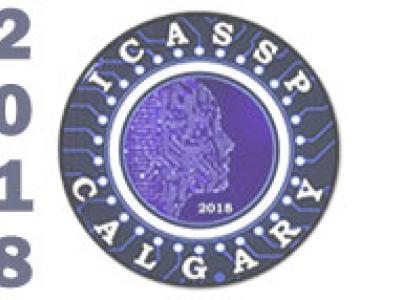
- Citation Author(s):
- Submitted by:
- Anh Pham
- Last updated:
- 25 April 2018 - 1:39pm
- Document Type:
- Presentation Slides
- Document Year:
- 2018
- Event:
- Presenters:
- Anh Pham
- Paper Code:
- 1818
- Categories:
- Log in to post comments
Multiple-instance learning is a framework for learning from data consisting of bags of instances labeled at the bag level. A common assumption in multi-instance learning is that a bag label is positive if and only if at least one instance in the bag is positive. In practice, this assumption may be violated. For example, experts may provide a noisy label to a bag consisting of many instances, to reduce labeling time. Here, we consider generalized multi-instance learning, which assumes that the bag label is non-deterministically determined based on the number of positive instances in the bag. The challenge in this setting is to simultaneous learn an instance classifier and the unknown bag-labeling probabilistic rule. This paper addresses the generalized multi-instance learning using a discriminative probabilistic graphical model with exact and efficient inference. Experiments on both synthetic and real data illustrate the effectiveness of the proposed method relative to other methods including those that follow the traditional multiple-instance learning assumption.