Documents
Poster
Attention-based Dialog State Tracking for Conversational Interview Coaching
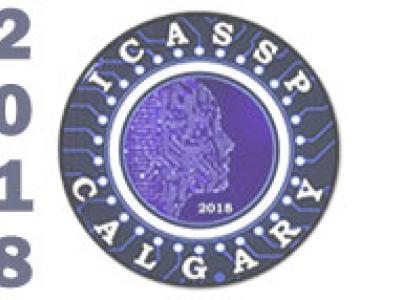
- Citation Author(s):
- Submitted by:
- Ming-Hsiang Su
- Last updated:
- 12 April 2018 - 11:49pm
- Document Type:
- Poster
- Document Year:
- 2018
- Event:
- Presenters:
- Ming-Hsiang Su
- Paper Code:
- HLT-P2.2
- Categories:
- Log in to post comments
This study proposes an approach to dialog state tracking (DST) in a conversational interview coaching system. For the interview coaching task, the semantic slots, used mostly in traditional dialog systems, are difficult to define manually. This study adopts the topic profile of the response from the interviewee as the dialog state representation. In addition, as the response generally consists of several sentences, the summary vector obtained from a long short-term memory neural network (LSTM) is likely to contain noisy information from many irrelevant sentences. This study proposes a sentence attention mechanism combining the sentence attention weights from a convolutional neural tensor network (CNTN) and the topic profile by selectively focusing on significant sentences for attention-based dialog state tracking. This study collected 260 interview dialogs consisting of 3,016 dialog turns for performance evaluation. A five-fold cross validation scheme was employed and the results show that the proposed method outperformed the semantic slot-based baseline method.