Documents
Poster
CONVOLUTIONAL SEQUENCE TO SEQUENCE MODEL WITH NON-SEQUENTIAL GREEDY DECODING FOR GRAPHEME TO PHONEME CONVERSION
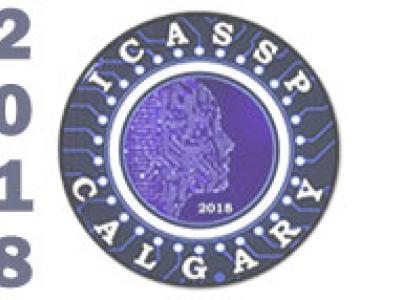
- Citation Author(s):
- Submitted by:
- Moon-jung Chae
- Last updated:
- 13 April 2018 - 12:22am
- Document Type:
- Poster
- Document Year:
- 2018
- Event:
- Presenters:
- Moon-jung Chae
- Paper Code:
- MLSP-P3.1
- Categories:
- Log in to post comments
The greedy decoding method used in the conventional sequence-to-sequence models is prone to producing a model with a compounding
of errors, mainly because it makes inferences in a fixed order, regardless of whether or not the model’s previous guesses are correct.
We propose a non-sequential greedy decoding method that generalizes the greedy decoding schemes proposed in the past. The proposed
method determines not only which token to consider, but also which position in the output sequence to infer at each inference step.
Specifically, it allows the model to consider easy parts first, helping the model infer hard parts more easily later by providing more information. We study a grapheme-to-phoneme conversion task with a fully convolutional encoder-decoder model that embeds the proposed
decoding method. Experiment results show that our model shows better performance than that of the state-of-the-art model in
terms of both phoneme error rate and word error rate.