Documents
Poster
DEEP CNN BASED FEATURE EXTRACTOR FOR TEXT-PROMPTED SPEAKER RECOGNITION
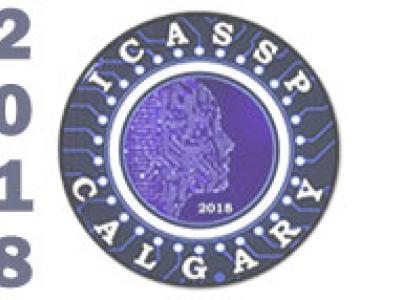
- Citation Author(s):
- Submitted by:
- Sergey Novoselov
- Last updated:
- 13 April 2018 - 5:08am
- Document Type:
- Poster
- Document Year:
- 2018
- Event:
- Paper Code:
- 2977
- Categories:
- Log in to post comments
Deep learning is still not a very common tool in speaker verification field. We study deep convolutional neural network performance in the text-prompted speaker verification task. The prompted passphrase is segmented into word states — i.e. digits — to test each digit utterance separately. We train a single high-level feature extractor for all states and use cosine similarity metric for scoring. The key feature of our network is the Max-Feature-Map activation function, which acts as an embedded feature selector. By using multitask learning scheme to train the high-level feature extractor we were able to surpass the classic baseline systems in terms of quality and achieved impressive results for such a novice approach, getting 2.85% EER on the RSR2015 evaluation set. Fusion of the proposed and the baseline systems improves this result.