Documents
Presentation Slides
Improved Algorithms for Differentially private Orthogonal Tensor Decomposition
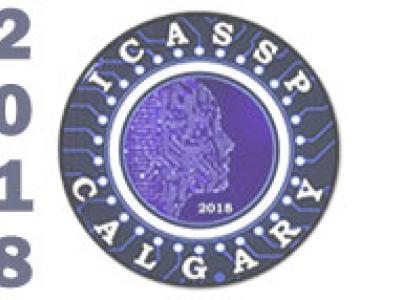
- Citation Author(s):
- Submitted by:
- Hafiz Imtiaz
- Last updated:
- 13 April 2018 - 2:23pm
- Document Type:
- Presentation Slides
- Document Year:
- 2018
- Event:
- Presenters:
- Hafiz Imtiaz
- Paper Code:
- 3345
- Categories:
- Log in to post comments
Tensor decompositions have applications in many areas including signal processing, machine learning, computer vision and neuroscience. In this paper, we propose two new differentially private algorithms for orthogonal decomposition of symmetric tensors from private or sensitive data; these arise in applications such as latent variable models. Differential privacy is a formal privacy framework that guarantees protections against adversarial inference. We investigate the performance of these algorithms with varying privacy and database parameters and compare against another recently proposed privacy-preserving algorithm. Our experiments show that the proposed algorithms provide very good utility even while preserving strict privacy guarantees.