Documents
Poster
Poster
A Random Matrix and Concentration Inequalities framework for Neural Networks Analysis
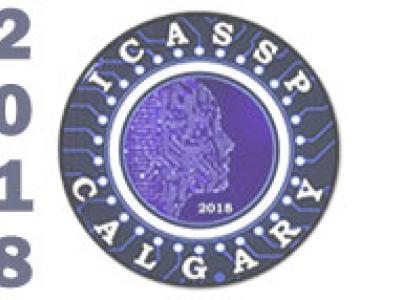
- Citation Author(s):
- Submitted by:
- Cosme Louart
- Last updated:
- 13 April 2018 - 5:38pm
- Document Type:
- Poster
- Document Year:
- 2018
- Event:
- Presenters:
- louart
- Paper Code:
- ICASSP18001
- Categories:
- Log in to post comments
Our article provides a theoretical analysis of the asymptotic performance of a regression or classification task performed by a simple random neural network. This result is obtained by leveraging a new framework at the crossroads between random matrix theory and the concentration of measure theory. This approach is of utmost interest for neural network analysis at large in that it naturally dismisses the difficulty induced by the non-linear activation functions, so long that these are Lipschitz functions. As an application, we provide formulas for the limiting law of the random neural network output and compare them conclusively to those obtained practically on handwritten digits databases.