Documents
Poster
RECURRENT NEURAL NETWORKS FOR AUTOMATIC REPLAY SPOOFING ATTACK DETECTION
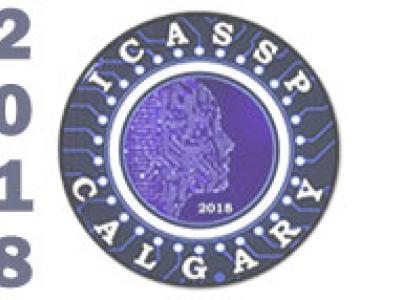
- Citation Author(s):
- Submitted by:
- Zhuxin Chen
- Last updated:
- 13 April 2018 - 10:28pm
- Document Type:
- Poster
- Document Year:
- 2018
- Event:
- Paper Code:
- IFS-P2.3
- Categories:
- Log in to post comments
In order to enhance the security of automatic speaker verification (ASV) systems, automatic spoofing attack detection, which discriminates the fake audio recordings from genuine human speech, has gain much attention recently. Among various ways of spoofing attacks, replay attacks are one of the most effective and economical methods. In this paper, we explore using recurrent neural networks for automatic replay spoofing attack detection. More specifically, we focus on recurrent neural networks with more sophisticated recurrent units that involve a gating mechanism, such as a long short term memory (LSTM) unit and a recently proposed gated recurrent unit (GRU). Our experimental results on the ASVspoof 2017 showed that neural networks significantly outperform Gaussian mixture models (GMM). In addition, we achieved the best equal error rate of 9.81% on the ASVspoof 2017 and 1.077% on the BTAS 2016 by using GRU models, which outperform the best feed-forward neural networks by 19% and 46%, relatively and respectively.