Documents
Presentation Slides
Unsupervised Domain Adaptation via Domain Adversarial Training for Speaker Recognition
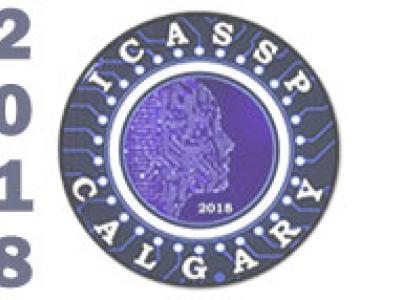
- Citation Author(s):
- Submitted by:
- Qing Wang
- Last updated:
- 25 April 2018 - 2:23am
- Document Type:
- Presentation Slides
- Document Year:
- 2018
- Event:
- Presenters:
- Qing Wang
- Paper Code:
- SP-L5.5
- Categories:
- Log in to post comments
The i-vector approach to speaker recognition has achieved good performance when the domain of the evaluation dataset is similar to that of the training dataset. However, in real-world applications, there is always a mismatch between the training and evaluation datasets, that leads to performance degradation. To address this problem, this paper proposes to learn the domain-invariant and speaker-discriminative speech representations via domain adversarial training. Specifically, with domain adversarial training method, we use a gradient reversal layer to remove the domain variation and project the different domain data into the same subspace. Moreover, we compare the proposed method with other state-of-the-art unsupervised domain adaptation techniques for the i-vector approach to speaker recognition (e.g. autoencoder based domain adaptation, inter dataset variability compensation, dataset-invariant covariance normalization, and so on).
Experiments on 2013 domain adaptation challenge (DAC) dataset demonstrate that the proposed method is not only effective in solving the dataset mismatch problem but also outperforms the compared unsupervised domain adaptation methods.