Documents
Poster
ICIP18001
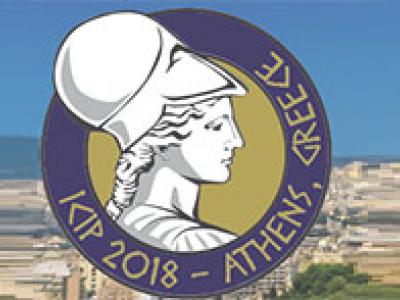
- Citation Author(s):
- Submitted by:
- Yiming Liang
- Last updated:
- 4 October 2018 - 11:02am
- Document Type:
- Poster
- Document Year:
- 2018
- Event:
- Presenters:
- Yiming Liang
- Paper Code:
- 1350
- Categories:
- Log in to post comments
Traditional methods for multiple object tracking usually consider features at image level and reason about simple space and time constraints. However, in this paper we propose a multiple object tracker based on LSTM network to learn temporally correlated features. Our tracker learns features on velocity, position and appearance aspects of the objects to improve tracking accuracy. In order to deal with occlusion problems, velocity model is also used to predict the lost detections for the occluded identities. Besides, pre-association is performed before appearance model to improve the efficiency of calculation. Final association problem is solved by taking the best match. Experiments in MOT17 dataset show that our tracker achieves leading performance compared to other state-of-the-art algorithms.
Index Terms— multiple object tracking, Long Short Term Memory, temporally correlated feature learning