Documents
Poster
How Should We Handle 4D Light Fields with CNNs?
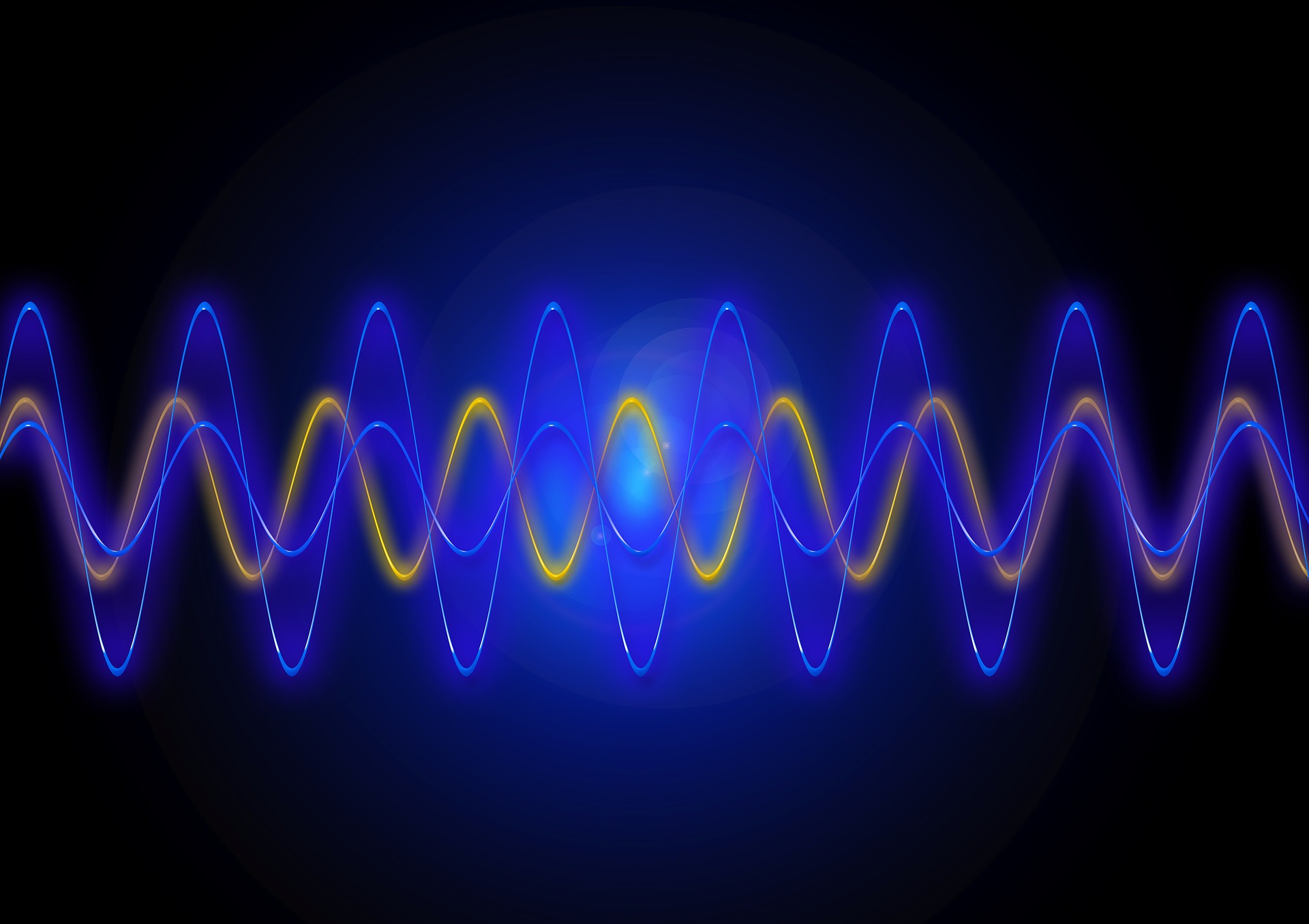
- Citation Author(s):
- Submitted by:
- Shu Fujita
- Last updated:
- 5 October 2018 - 12:39am
- Document Type:
- Poster
- Categories:
- Log in to post comments
We investigated how we should handle high dimensional light fields (LFs) with convolutional neural networks (CNNs).
An LF is a 4-D signal representation of light rays, and it is interpreted as a set of dense multi-view images.
As an important building block of various light field applications, we focused on signal restoration problems for LFs, and we adopted CNNs as the solver for them because of its striking performance on the conventional 2-D images.
In applying CNNs, the high dimensionality of LFs should be carefully addressed.
Instead of treating the full 4-D signal as it is, we followed a divide and conquer strategy.
Specifically, we cascade two or three CNNs, each of which works only on 2-D subspace of the full 4-D LFs.
Combining CNNs that work on different subspaces, we can eventually handle the full 4-D structure.
Moreover, considering different properties of those subspaces, we experimentally explored the best
combination in which different subspaces are cascaded.
Although our experiments are currently limited to a denoising problem, the lessons found from our results will benefit the prospective research on the full 4-D LF processing.