Documents
Presentation Slides
Tensor Ensemble Learning
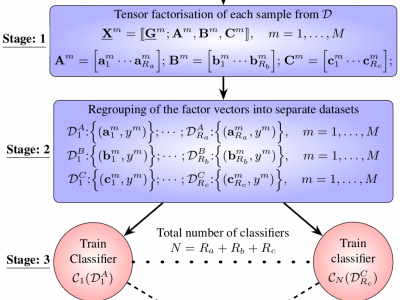
- Citation Author(s):
- Submitted by:
- ILYA KISIL
- Last updated:
- 23 November 2018 - 1:09pm
- Document Type:
- Presentation Slides
- Document Year:
- 2018
- Event:
- Presenters:
- Ilia Kisil, Ahmad Moniri, Danilo P. Mandic
- Paper Code:
- GLOBALSIP18001
- Categories:
- Keywords:
- Log in to post comments
In big data applications, classical ensemble learning is typically infeasible on the raw input data and dimensionality reduction techniques are necessary. To this end, novel framework that generalises classic flat-view ensemble learning to multidimensional tensor- valued data is introduced. This is achieved by virtue of tensor decompositions, whereby the proposed method, referred to as tensor ensemble learning (TEL), decomposes every input data sample into multiple factors which allows for a flexibility in the choice of multiple learning algorithms in order to improve test performance. The TEL framework is shown to naturally compress multidimensional data in order to take advantage of the inherent multi-way data structure and exploit the benefit of ensemble learning. The proposed framework is verified through the application of Higher Order Singular Value Decomposition (HOSVD) to the ETH-80 dataset and is shown to outperform the classical ensemble learning approach of bootstrap aggregating.