Documents
Poster
Poster of pixel level data augmentation for semantic image segmentation using generative adversarial networks
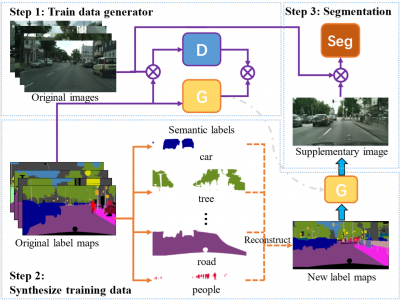
- Citation Author(s):
- Submitted by:
- Jiaqi Zhang
- Last updated:
- 8 May 2019 - 7:18am
- Document Type:
- Poster
- Document Year:
- 2019
- Event:
- Presenters:
- Jiaqi Zhang
- Paper Code:
- 4496
- Categories:
- Log in to post comments
Semantic segmentation is one of the basic topics in computer vision, it aims to assign semantic labels to every pixel of an image. Unbalanced semantic label distribution could have a negative influence on segmentation accuracy. In this paper, we investigate using data augmentation approach to balance the label distribution in order to improve segmentation performance. We propose using generative adversarial networks (GANs) to generate realistic images for improving the performance of semantic segmentation networks. Experimental results show that the proposed method can not only improve segmentation accuracy of those classes with low accuracy,but also obtain 1.3% to 2.1% increase in average segmentation accuracy. It proves that this augmentation method can boost the accuracy and be easily applicable to any other segmentation models.