Documents
Poster
Poster
Minimum-Volume Rank-Deficient Nonnegative Matrix Factorizations
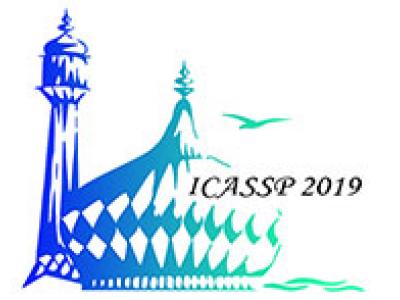
- Citation Author(s):
- Submitted by:
- Valentin Leplat
- Last updated:
- 8 May 2019 - 9:58am
- Document Type:
- Poster
- Document Year:
- 2019
- Event:
- Presenters:
- Valentin Leplat
- Paper Code:
- 1108
- Categories:
- Log in to post comments
In recent years, nonnegative matrix factorization (NMF) with volume regularization has been shown to be a powerful identifiable model; for example for hyperspectral unmixing, document classification, community detection and hidden Markov models. We show that minimum-volume NMF (min-vol NMF) can also be used when the basis matrix is rank deficient, which is a reasonable scenario for some real-world NMF problems (e.g., for unmixing multispectral images). We propose an alternating fast projected gradient method for minvol NMF and illustrate its use on rank-deficient NMF problems; namely a synthetic data set and a multispectral image.