Documents
Poster
A DENOISING AUTOENCODER FOR SPEAKER RECOGNITION. RESULTS ON THE MCE 2018 CHALLENGE
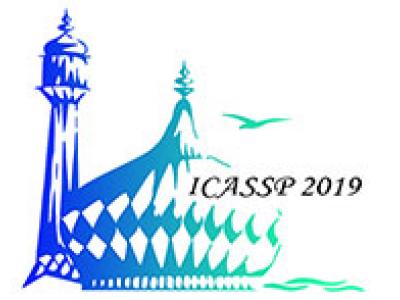
- Citation Author(s):
- Submitted by:
- Roberto Javier ...
- Last updated:
- 9 May 2019 - 5:56am
- Document Type:
- Poster
- Document Year:
- 2019
- Event:
- Presenters:
- Roberto Font
- Paper Code:
- 1809
- Categories:
- Log in to post comments
We propose a Denoising Autoencoder (DAE) for speaker recognition, trained to map each individual ivector to the mean of all ivectors belonging to that particular speaker. The aim of this DAE is to compensate for inter-session variability and increase the discriminative power of the ivectors prior to PLDA scoring. We test the proposed approach on the MCE 2018 1st Multi-target speaker detection and identification Challenge Evaluation. This evaluation presents a call-center fraud detection scenario: given a speech segment, detect if it belongs to any of the speakers in a blacklist. We show that our DAE system consistently outperforms the usual LDA + PLDA pipeline, achieving a Top-S EER of 4.33% and Top-1 EER of 6.11% on the evaluation set, which represents a 45.6% error reduction with respect to the baseline system provided by organizers.