Documents
Poster
Improving Graph Trend Filtering with Non-Convex Penalties
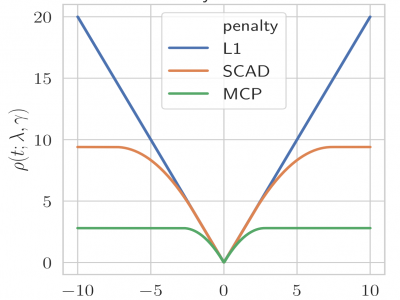
- Citation Author(s):
- Submitted by:
- Harlin Lee
- Last updated:
- 9 June 2019 - 8:24pm
- Document Type:
- Poster
- Document Year:
- 2019
- Event:
- Presenters:
- Harlin Lee
- Paper Code:
- SPTM-P9.5
- Categories:
- Keywords:
- Log in to post comments
In this paper, we study the denoising of piecewise smooth graph sig-nals that exhibit inhomogeneous levels of smoothness over a graph. We extend the graph trend filtering framework to a family of non-convex regularizers that exhibit superior recovery performance overexisting convex ones. We present theoretical results in the form ofasymptotic error rates for both generic and specialized graph models. We further present an ADMM-based algorithm to solve the proposedoptimization problem and analyze its convergence. Numerical per-formance of the proposed framework with non-convex regularizerson both synthetic and real-world data are presented for denoising,support recovery, and semi-supervised classification. https://ieeexplore.ieee.org/abstract/document/8683279