Documents
Poster
A Multi-Task Bayesian Deep Neural Net for Detecting Life-Threatening Infant Incidents From Head Images
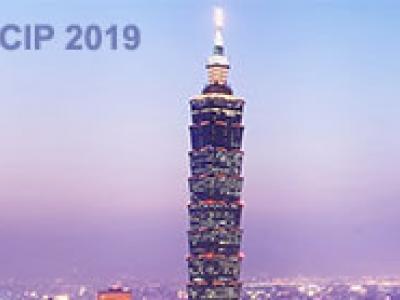
- Citation Author(s):
- Submitted by:
- Tzu-Jui Julius Wang
- Last updated:
- 19 September 2019 - 8:54am
- Document Type:
- Poster
- Document Year:
- 2019
- Event:
- Presenters:
- Julius Wang and Yi-Ping Liao
- Categories:
- Log in to post comments
The notorious incident of sudden infant death syndrome (SIDS) can easily happen to a newborn due to many environmental factors. To prevent such tragic incidents from happening, we propose a multi-task deep learning framework that detects different facial traits and two life-threatening indicators, i.e. which facial parts are occluded or covered, by analyzing the infant head image. Furthermore, we extend and adapt the recently developed models that capture data-dependent uncertainty from noisy observations for our application. The experimental results show significant improvements on YunInfants dataset across most of the tasks over the models that simply adopt the regular cross-entropy losses without addressing the effect of the underlying uncertainties.