Documents
Poster
REINFORCING THE ROBUSTNESS OF A DEEP NEURAL NETWORK TO ADVERSARIAL EXAMPLES BY USING COLOR QUANTIZATION OF TRAINING IMAGE DATA
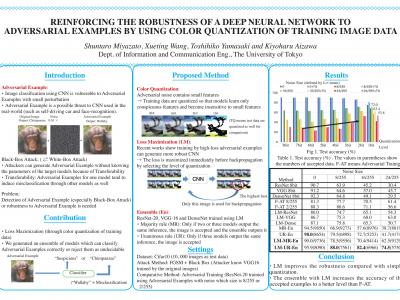
- Citation Author(s):
- Submitted by:
- Shuntaro Miyazato
- Last updated:
- 20 September 2019 - 11:33am
- Document Type:
- Poster
- Document Year:
- 2019
- Event:
- Presenters:
- Shuntaro Miyazato
- Paper Code:
- 3045
- Categories:
- Log in to post comments
Recent works have shown the vulnerability of deep convolu-tional neural network (DCNN) to adversarial examples withmalicious perturbations. In particular, Black-Box attackswithout information of parameter and architectures of thetarget models are feared as realistic threats. To address thisproblem, we propose a method using an ensemble of mod-els trained by color-quantized data with loss maximization.Color-quantization can allow the trained models to focuson learning conspicuous spatial features to enhance the ro-bustness of DCNNs to adversarial examples. The proposedmethod can be adapted to Black-Box attacks with no needof particular attack algorithm for the defense. The resultsof our experiments validated the effectiveness for preventingdecrease in the test accuracy with adversarial perturbation.